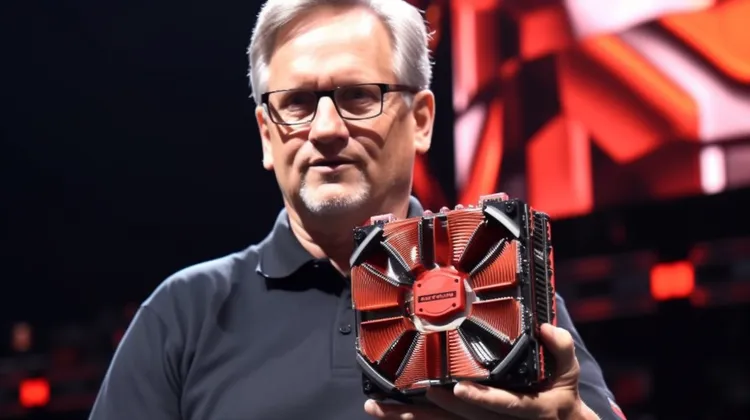
AMD’s AI chip challenge
Artificial intelligence (AI) is one of the most talked-about technologies globally, capable of impacting the way we live and work. There are countless applications of AI, ranging from self-driving cars to intelligent chatbots and personalized marketing campaigns. However, the development of AI technologies requires advanced hardware, and that’s where AMD’s new chip comes in. AMD has recently launched its Radeon Instinct MI100 GPU, which is fast becoming a popular choice for AI workloads and machine learning applications.
The Radeon Instinct MI100 GPU is a highly powerful AI chip designed to compete with Nvidia’s dominant range of graphics processors. AMD has been working towards this milestone for years, and the MI100 is the perfect testament to its efforts. The Radeon Instinct MI100 is built on the advanced CDNA (Compute DNA) architecture, which allows it to deliver high performance and power efficiency. The architecture has been optimized for HPC (High-Performance Computing) workloads, making it ideal for AI and machine learning.
One of the most appealing features of the MI100 GPU is its ability to carry out both training and inference tasks. The training process is essential for training AI models, and it requires massive computing power. Inference, on the other hand, is the process of executing the trained model and is generally less resource-intensive. For a long time, AI developers had to use different hardware components to carry out these tasks, but with the MI100, it can be done on a single chip. This significantly improves the performance and efficiency of AI workloads.
With the MI100 GPU, AMD aims to challenge Nvidia’s domination in the AI hardware market, which is currently focused mainly on Nvidia’s Tesla GPUs. The MI100 GPU’s biggest advantage over the Tesla GPUs is its open ecosystem. While Nvidia’s hardware is fantastic at delivering performance, it’s heavily tied to proprietary software. The open-source nature of the MI100 GPU allows AI developers to leverage the power of the ecosystem by using their preferred software tools. This open ecosystem approach makes it easier for AI developers to access cutting-edge AI technology, ultimately leading to a faster pace of innovation.
Another benefit that the MI100 has over the Tesla GPUs is HBM2 (High Bandwidth Memory) technology. HBM2 helps to overcome the memory bandwidth bottleneck and is particularly useful when working with large datasets. With its massive 32GB of HBM2 memory, the MI100 GPU can handle the most demanding AI workloads, something that the Tesla GPUs may struggle with.
However, it’s worth mentioning that Nvidia has been the market leader in the AI hardware space for a long time, and dethroning it won’t be easy. Nvidia has built a formidable reputation for delivering robust AI hardware and software stacks that are favored by data scientists and AI developers globally. Nonetheless, with the current features of the MI100 GPU, AMD has gotten off to a good start in the AI chip market, and there’s plenty of room for growth. AMD and Nvidia’s products are comparable in performance and suitability, and ultimately, it might come down to price to strike a balance.
Another factor that could sway developers towards the MI100 GPU is the availability of exclusive machine learning software designed for the chip. AMD has already started making significant strides with software developers, illustrating how easy it is to access the MI100’s computing power. The software allows for intricacies of machine learning to be optimized for the hardware, creating a more efficient exchange of data between the chip and other computing components in a system. In the long run, dedicated software like this could make a big difference in the speed and efficiency of AI training and inference.
The MI100 GPU supports a broad range of AI libraries such as TensorFlow, PyTorch, and Caffe, making it easy for developers to integrate their existing deep learning models with the MI100 ecosystem. Also, AMD has put significant effort into developing an easy-to-use development kit, making it easy for developers to use the chip’s capabilities.
Furthermore, the MI100 GPU’s power efficiency also makes it highly attractive, especially with energy efficiency and IT costs becoming a significant point of focus for large organizations. The MI100 requires less energy to perform AI workloads, making it highly cost-effective, and in the long run, beneficial for both organizations and the environment.
Overall, the Radeon Instinct MI100 GPU is shaping up to be a worthy competitor for Nvidia’s AI hardware. Though Nvidia has a considerable market share and a solid reputation for delivering excellent hardware and software ecosystems, the MI100 stands up well. AMD’s open ecosystem, HBM2 technology, energy efficiency, and exclusive machine learning software are excellent selling points for data scientists and AI developers. It’s now left to see how well the MI100 GPU performs in actual AI applications, and if it will gain ground on Nvidia’s Tesla GPUs.
10 thoughts on “AMD’s AI chip challenge”
Leave a Reply
You must be logged in to post a comment.
The MI100 GPU may have HBM2 technology, but that alone doesn’t guarantee superior performance compared to Nvidia’s Tesla GPUs. Nvidia has a long history of delivering cutting-edge technology and staying ahead of the competition. Will AMD be able to keep up?
While the MI100 GPU may offer energy efficiency, it’s important to remember that Nvidia’s Tesla GPUs have already proven their performance and reliability. Organizations may prioritize performance over energy efficiency when it comes to AI workloads. β‘
Overall, the MI100 GPU seems like a worthy competitor for Nvidia’s AI hardware. Its features and capabilities are impressive, and I’m excited to see how it performs in real-world AI applications. Go AMD! ππ
The open ecosystem approach of the MI100 GPU is a game-changer. It gives AI developers the freedom to use their preferred software tools and promotes a faster pace of innovation. πβ¨
While the MI100 GPU may have its strengths, it’s important to question whether it can truly match the capabilities and reputation of Nvidia’s hardware and software stacks. Nvidia has become the go-to choice for many data scientists and AI developers for a reason. π
I doubt that the MI100 GPU will be able to compete with Nvidia’s dominance in the AI hardware market. Nvidia has established itself as the leader in this field, and it will be a tough challenge for AMD to dethrone them.
I question whether the MI100 GPU’s exclusive machine learning software will truly be advantageous. Nvidia has already established itself as a leader in the field, and many developers are already familiar with their software stacks. It’s a tough market to break into.
While the MI100 GPU may have its strengths, it’s important to remember that Nvidia has a long history of delivering excellent hardware and software ecosystems tailored specifically for AI workloads. AMD will need to do more to convince developers to switch.
Energy efficiency is a major concern, and it’s great to see the MI100 GPU being highly power-efficient. It’s a win-win for organizations and the environment!
While the MI100 GPU may offer an open ecosystem, it remains to be seen if it can truly provide the same level of performance and compatibility as Nvidia’s Tesla GPUs. Developers may be hesitant to switch to a less established player in the market. π